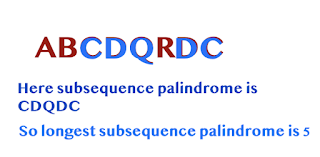
Longest palindrome Sub-sequence from the given String using Dynamic Programming
Write a program to find the longest sub-sequence palindrome from the given string by using dynamic programming. For example
Input String : ABCDQRDC
Longest sub-sequence palindrome: 5
Input String : ABCDQRDC
Longest sub-sequence palindrome: 5
- So let's see how we will solve and find the longest subsequence palindrome using dynamic programming.
- Construct the solution matrix of size NxN where N is the length of given string.
- We will calculate the size of each character sequence palindrome size and will the memorised in the solution matrix which will referred for the next incremental sequence.
- For example in above example all strings of 1 character will be palindrome size of 1. A, B, C, D, Q, R, D, C
- On next cycle it will add another character and makes 2 character string like
- AB, BC, CD, DQ, QR, RD, DC and goes on like
- ABC, BCD, CDQ, DQR, QRD, RDC etc.,
- Each sequences palindrome size will be stored in the solution array and finally returns the maximum size as output.
Now lets see simple Java code
public class LongestPalindromeSequence { public static void main(String[] args) { String str = "ABCDQRDC"; LongestPalindromeSequence obj = new LongestPalindromeSequence(); System.out.println("\nLength of Longest palindrome subsequence : " + obj.lps(str)); } public int lps(String seq) { int n = seq.length(); int j, k, i; int L[][] = new int[n][n]; // Strings of 1 character will be palindrome size of 1 // starting with upper matrix and all single character falls diagonally and size will be 1 for (j = 0; j < n; j++) L[j][j] = 1; for (i = 2; i <= n; i++) { for (j = 0; j < n - i + 1; j++) { k = j + i - 1; if (seq.charAt(j) == seq.charAt(k)) { L[j][k] = L[j + 1][k - 1] + 2; } else { L[j][k] = Math.max(L[j][k - 1], L[j + 1][k]); } } } /*System.out.println("Final solution Matrix :::: "); for (j = 0; j < n; j++) { System.out.print(seq.charAt(j) +" - "); for (k = 0; k < n; k++) { System.out.print(L[j][k] + "\t"); } System.out.println(); }*/ return L[0][n - 1]; } }
OUTPUT:
Length of Longest palindrome subsequence : 5